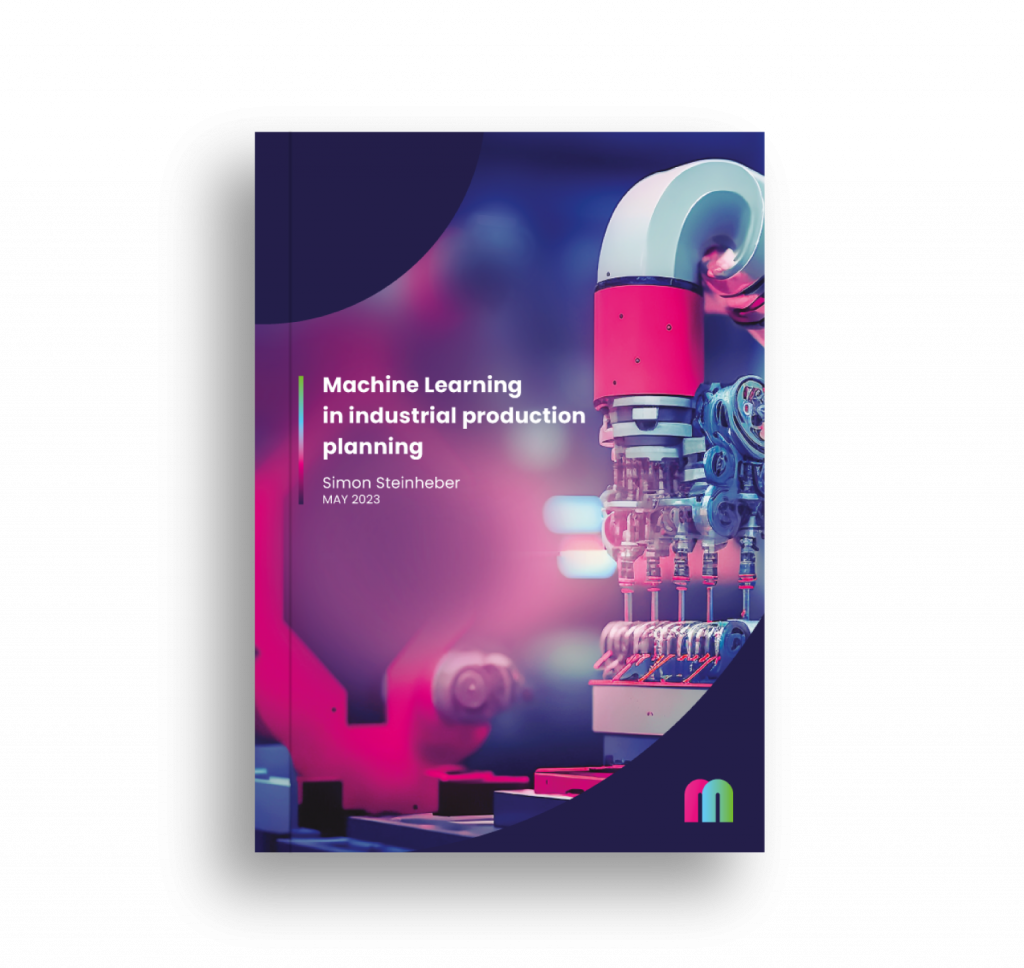
In our whitepaper for free download, MLOps Engineer Simon Steinheber shows how machine learning works in industrial production planning.
“When do we know that model training is complete and we can deploy the finished model?” Many data scientists are familiar with this question. After improving model quality in numerous experiments in Jupyter Notebooks they need to make the code and model artifacts production-ready.
This is what you take with you:
- How can this transition be made stress-free?
- What role does Jupyter Notebooks play in productive machine learning?
- Why should you be suspicious of the question asked at the beginning?
Reducing production costs through machine learning
Storage costs represent a significant expense in industrial production for companies. An AI platform capable of predicting future product demand can offer a pathway to optimizing these costs.
The objective of our project was to generate accurate predictions for an initial set of 100 different products in an initial facility.